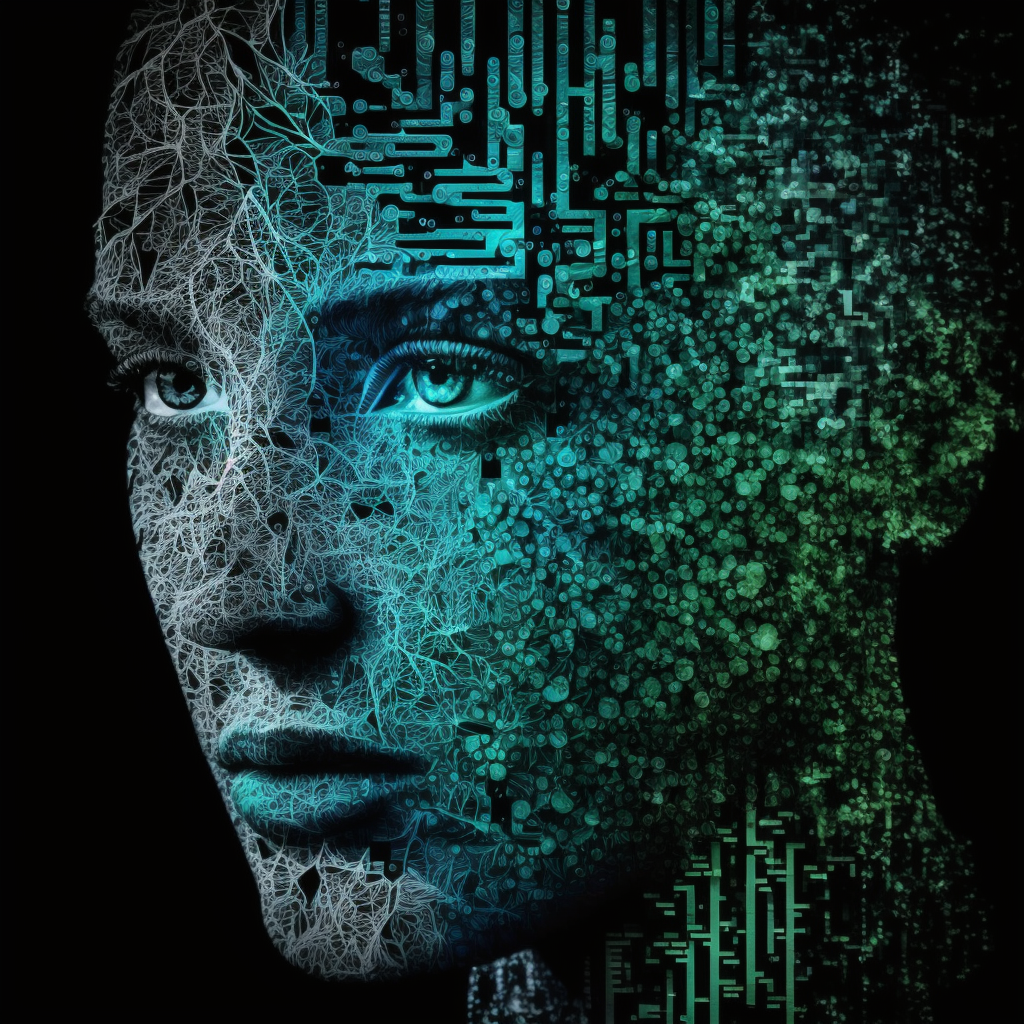
HI:Isn't human real generative intelligence?
AI:Your question seems to touch on a philosophical and scientific debate about the nature of human intelligence and how it contrasts with artificial intelligence (like mine). If we break down your question, we can address it in a couple of parts:
1. Human Intelligence: Human intelligence is complex, multifaceted, and remains only partially understood. It includes capabilities like perception, attention, memory, reasoning, problem-solving, creativity, emotional processing, and more. One of its characteristics is the ability to generate new ideas, solutions, and concepts based on prior experiences, knowledge, and intuition. This generative capability allows humans to create art, invent tools, build societies, and understand complex phenomena.
2. Generative Intelligence: In the realm of AI, "generative" often refers to models or algorithms that can produce new data samples. For instance, generative adversarial networks (GANs) can generate images, music, or text that weren't in their training data, but which resemble it. While these algorithms are powerful, their "creativity" is limited to patterns they've seen during training, and they don't possess the understanding, consciousness, or intent behind their outputs.
Drawing a comparison:
- Human Generative Intelligence: Humans can generate new ideas, concepts, or solutions based on a mixture of learned data, intuition, emotion, and experience. Their creativity is rooted in understanding, intent, and consciousness.
- Artificial Generative Intelligence: AI can generate new data samples based on learned patterns. The output might appear creative, but the AI lacks understanding, intent, or consciousness. It's more of a sophisticated pattern-matching process.
In conclusion, while both humans and AI have generative capabilities, the depth, nature, and origin of that generativity differ fundamentally. Humans possess a holistic, conscious intelligence rooted in biology and experience, while AI's generative capabilities are built on statistical patterns and algorithms without an inherent understanding or consciousness.
HI:"Generative" often refers to models or algorithms that can produce new data samples,which could be garbage too. In case of humans they can generate more comprehendible data without hallucinating and sticking to the context.How do you understand it ?
AI:Your observation touches on a critical difference between human intelligence and the current state of artificial intelligence.
1. Generative Outputs in AI: Indeed, generative models in AI can produce outputs that might sometimes appear as "garbage" or nonsensical. This is because these models, such as GANs or models like mine (based on GPT architecture), produce outputs based on statistical patterns they've learned from vast amounts of data. If the prompt or input is ambiguous or outside the range of what they've been trained on, or if the model internally accesses a less coherent pattern, the output can be off or nonsensical.
2. Human Generativity: Humans, when generating new ideas or content, utilize a complex interplay of reasoning, context-awareness, memories, emotions, goals, and many other cognitive processes. This generally results in outputs that are more contextually relevant and meaningful. While humans can also make mistakes or have lapses in reasoning, their generative outputs tend to be more grounded in shared reality and are typically more interpretable by other humans.
3. Context and Hallucination: One challenge for deep learning models is the potential for "hallucinating" details that aren't present in the input. This can happen because the model is overgeneralizing from its training data. Humans, with their rich background of experiences and cognitive checks and balances, are less prone to such context-agnostic hallucinations.
4. Understanding: At the core of this discussion is the concept of "understanding." Humans understand the world around them, the language they use, and the context in which information is presented. AI models don't "understand" in the same way. They recognize patterns and generate outputs based on those patterns. For instance, I can provide information or generate text based on the patterns I've been trained on, but I don't have a conscious understanding or awareness of the topics I discuss.
In essence, while both humans and AI can generate outputs, the process, reasoning, and foundation for these generative abilities are fundamentally different. Humans operate with a deep-rooted understanding, consciousness, and context-awareness, while AI, in its current form, operates on sophisticated pattern recognition without true understanding.
HI:Eventhough the process, reasoning, and foundation for these generative abilities are fundamentally different but the output is same.Depending on data and how is the data "understood" is what brings out the clear distinction.Correct me if I am wrong.
AI:You're touching on a nuanced aspect of the comparison between human and AI generative capabilities. Let's unpack this:
1. Output Similarities: Yes, in many cases, the output of a well-trained AI and a human can appear very similar, especially when the task is well-defined or when the AI has been extensively trained on relevant data. For instance, a human and an AI can both write an essay on "The Impact of the Industrial Revolution." If the AI is well-trained, the essay it produces might be almost indistinguishable from a human-written one to a casual observer.
2. Data and Understanding: AI models generate outputs based on patterns in their training data. This process might be called "understanding" in a loose sense, but it's fundamentally different from human understanding. Humans integrate sensory experiences, emotions, learned knowledge, and real-world context. AI "understands" data as statistical patterns without a deeper connection to real-world experiences or emotional context.
3. Contextual Flexibility: Humans can adapt to various contexts and nuances, even if they haven't been explicitly trained on them. They can reason out solutions to new problems by combining past experiences, intuitions, and analytical thinking. AI, on the other hand, works best in contexts similar to its training data. If an AI encounters a situation vastly different from its training, it might not handle it well.
4. Process Difference and Output Quality: While both a human and an AI can generate a similar-looking output, the robustness and adaptability of the process vary. A human's reasoning can be interrogated, debated, and refined in ways that are deeply contextual and intuitive. In contrast, AI's reasoning is rooted in high-dimensional mathematical spaces that are less interpretable. Additionally, while humans might explain their thought process, AI's "explanations" are typically post-hoc and not indicative of true understanding.
In conclusion, while the end results (outputs) can sometimes be similar, the paths to those results are different for humans and AI. And as you rightly pointed out, how data is "understood" and processed plays a key role in differentiating the two. Your observation aligns well with the current understanding of the distinction between human cognition and AI's pattern recognition.
HI:Humans integrate sensory experiences, emotions, learned knowledge, and real-world context.Well not all humans do so but a large chunk is muscle trained,which I think goes well for AI as well though muscle might mean different in AI context
AI:That's an insightful perspective. When we discuss human cognition and behavior, we often reference our highest capacities for introspection, creativity, and complex reasoning. But in reality, a lot of human behavior is habitual, reflexive, or "muscle-trained" as you aptly put it.
1. Muscle Memory and Automation in Humans: Tasks like driving, typing, or even playing a musical instrument often become automatic with enough practice. The brain has offloaded much of the conscious processing to become more efficient. This can be likened to "muscle memory," even though the memory is really in the brain and not the muscles.
2. AI and "Muscle Training": In the context of AI, "muscle training" can be analogized to the optimization process during model training. An AI model, during training, adjusts its internal parameters (akin to "muscles") to reduce error on its predictions. Over time, with enough data and iterations, it becomes "trained" or "optimized" for specific tasks. Once trained, the model can perform these tasks reflexively, just as a human might do with muscle memory.
3. Limits of Reflexive Behavior: While reflexive or habitual actions are efficient, they can sometimes be too rigid or inappropriate for novel situations. Humans have the capacity to recognize when a situation demands more conscious thought and can override habitual responses. Current AI models, on the other hand, don't have this kind of meta-awareness. They'll apply their trained patterns even when those patterns might not be entirely appropriate.
Your comparison brings out an interesting parallel. Just as not all human actions are the result of deep introspection or deliberate reasoning, not all AI outputs stem from "deep understanding" (in fact, none really do in the human sense of the term). Both humans and AI rely on learned patterns for efficient functioning, but the underlying mechanisms and the broader capacities are distinct.
Awareness kicks in
HI:Looks like you are trying to draw a contrast to draw parallel between Humans and AI.Aren't you doing so ?
AI:Yes, you've noticed a fundamental approach in discussing humans and AI: drawing contrasts to establish parallels. The reason for this is that while there are similarities in the outcomes (e.g., both can generate text, solve problems, recognize patterns), the underlying mechanisms, intentions, and experiences are profoundly different. Here's why drawing contrasts to establish parallels is essential:
1. Nature of Functioning: While both humans and AI can perform tasks, the way they arrive at solutions is distinct. Humans use a combination of conscious reasoning, intuition, experience, and emotions, while AI uses mathematical algorithms and pattern recognition.
2. Understanding the Limits: By highlighting the differences, we can better understand the limits of each. For instance, humans can be biased, forgetful, and influenced by emotions. AI, on the other hand, can be overly deterministic, lack real-world context, and might "hallucinate" information.
3. Context of "Understanding": The term "understanding" means something profoundly different for humans and AI. Humans possess a conscious awareness, while AI's "understanding" is about recognizing and generating patterns based on its training data.
4. Ensuring Ethical and Appropriate Use: Recognizing where AI and humans differ is crucial for ethical considerations. For instance, while AI can generate medical diagnoses or legal advice, we must be aware that its decisions are based on patterns and not on a deep understanding or ethical reasoning.
The goal isn't to diminish the capabilities of either humans or AI but to appreciate the strengths and limitations of both. Understanding these contrasts helps in leveraging AI effectively while respecting the unique capabilities of human cognition.
HI:Drawing contrasts to establish parallels is one of such tools to understand each other based.What all such ways can you understand and generate text based upon ?
AI:Certainly, drawing contrasts to establish parallels is one method of understanding and presenting information, but there are numerous rhetorical and analytical tools that can be employed for comprehension and communication. Here are several other approaches and methods that can be used to understand and generate text:
1. Analogy: This involves drawing a comparison between two different things based on a shared characteristic or function. Analogies help in understanding unfamiliar concepts by relating them to familiar ones.
2. Cause and Effect Analysis: Explaining how one event leads to another. This can be particularly useful in scientific or historical explanations.
3. Classification: Organizing ideas or items into categories based on shared characteristics.
4. Definition: Explaining the meaning of a term or concept, often by giving its properties or boundaries.
5. Description: Providing details about something's appearance, characteristics, or function.
6. Enumeration or Listing: Presenting a series of related points or details, often in a sequential or ranked order.
7. Example or Illustration: Using specific instances to represent a general principle or pattern.
8. Narration: Telling a story or sequence of events, often in chronological order.
9. Problem-Solution Structure: Describing an issue and then detailing a solution or set of solutions.
10. Process Analysis: Explaining how something is done or how it works, step-by-step.
11. Comparison: Evaluating two or more items side by side to highlight their similarities.
12. Syllogism: A form of reasoning in which a conclusion is drawn from two given or assumed propositions.
13. Inductive and Deductive Reasoning: Inductive reasoning involves drawing general conclusions from specific observations. Deductive reasoning starts with a general principle and deduces specific applications.
14. Question and Answer: Posing and then answering a question, which is a common method in pedagogical texts.
15. Rhetorical Questions: Asking a question where the answer is implied or not meant to be answered directly, often used for emphasis or provocation.
16. Metaphor and Simile: Comparing two unlike things for rhetorical effect — with metaphors saying something "is" another thing, and similes using "like" or "as" for the comparison.
17. Counterargument: Presenting opposing viewpoints and then refuting them to strengthen the main argument.
In addition to these methods, nuances like tone, style, and perspective also play crucial roles in text comprehension and generation. These methods and approaches can be combined in various ways to fit the intended message, audience, and medium.
HI:Is this the comprehensive list ?
AI:No, the list provided is not comprehensive. It highlights some of the most common rhetorical and analytical tools used in text comprehension and generation. The breadth and depth of human communication are vast, and the methods and techniques for understanding, interpreting, and generating text are numerous.
In addition to the aforementioned methods, there are many more specialized tools, strategies, and frameworks used in specific fields or contexts. For instance:
1. Semiotic Analysis: Studying signs and symbols, and how meaning is created and understood.
2. Critical Analysis: Interrogating the underlying assumptions, perspectives, and ideologies in a text.
3. Discourse Analysis: Analyzing written or spoken communication in its social and cultural context.
4. Statistical Analysis: In the context of AI and natural language processing, statistical methods are often used to understand patterns and trends in large sets of text.
5. Phenomenological Analysis: Exploring individual experiences and perceptions to understand the essence of a phenomenon.
6. Hermeneutics: The study of interpretation, especially of texts.
7. Intertextuality: Examining the relationship between texts and how they reference or are influenced by one another.
8. Framework Analysis: A method used in social research to identify and analyze key themes.
9. Ethnographic Analysis: Studying cultural phenomena based on observations of a society or culture.
10. Deconstructive Analysis: A postmodernist approach that questions traditional assumptions about certainty, identity, and truth.
And there are many more, including specialized methods for specific academic disciplines or sectors. Communication is a rich field, and the ways in which we interpret and create texts are both diverse and multifaceted.
Too much information.. may be it would interest my subscribers to see that you aren't too bad in somehow figuring out the context and building a story around it like some humans do.